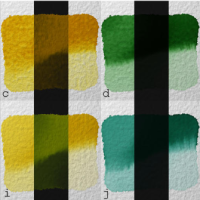
Aiding watercolor paintings
These are some notes on things I learned while researching the topic of watercolor simulation for a project I worked on. Due to the nature of the project I can’t provide specifics or any source code this time, but I think the basics of the problem make for an interesting topic to write about. Image Read More …